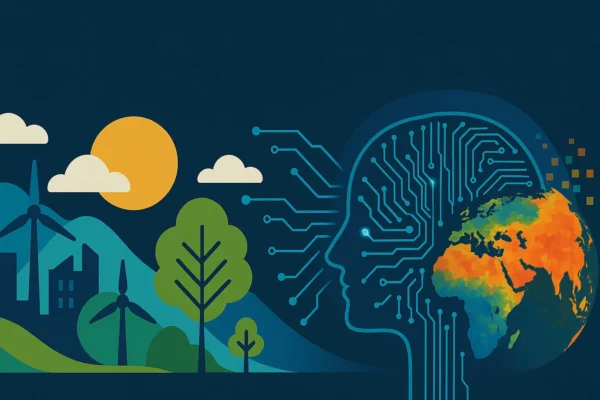
Discover how generative AI is revolutionizing climate modeling with faster, high-resolution simulations and accurate weather predictions. Explore real-world applications, benefits, challenges, and the future of AI in climate science as of April 2025
Climate modeling is critical for predicting future climate scenarios, informing disaster preparedness, and guiding global policy to mitigate climate change. Traditional models, grounded in physics-based simulations, excel at capturing Earth’s complex systems but face significant limitations: they are computationally intensive, often requiring supercomputers, and can take weeks to generate long-term projections. Additionally, they struggle with incomplete datasets and high uncertainty in extreme weather predictions.
Generative AI, a transformative technology that leverages algorithms to create realistic data, offering a paradigm shift. By accelerating simulations, enhancing resolution, and filling data gaps, generative AI is redefining climate modeling, enabling faster and more actionable insights.
What Is Generative AI and How Does It Work?
Generative AI refers to algorithms, such as generative adversarial networks (GANs), diffusion models, and transformers, that generate new data resembling their training sets. GANs pit two neural networks against each other—a generator creating data and a discriminator evaluating its authenticity—producing highly realistic outputs. Diffusion models, like those powering advanced image generation, iteratively refine noisy data into coherent patterns, while transformers excel at processing sequential data for predictions. In climate modeling, these capabilities enable the simulation of complex climate scenarios, recognition of intricate atmospheric patterns, and generation of synthetic datasets to augment sparse observations, making generative AI a powerful tool for environmental forecasting.
 Top Applications of Generative AI in Climate Modeling:
Generative AI is revolutionizing climate science through diverse applications:
- High-Resolution Climate Simulations: AI models produce detailed climate projections, capturing fine-scale phenomena like regional temperature shifts or precipitation patterns, previously computationally prohibitive.
- Predicting Extreme Weather Events: By analyzing historical and real-time data, AI enhances forecasts for hurricanes, heatwaves, and floods, improving early warning systems.
- Generating Synthetic Climate Scenarios: Generative AI creates plausible future climate scenarios, aiding policymakers in stress-testing adaptation strategies.
- Enhancing Satellite Data and Missing Data Reconstruction: AI reconstructs incomplete datasets from satellites or historical records, filling gaps in sparse regions like the Arctic or developing nations.
These applications empower scientists to model climate dynamics with unprecedented precision and speed.
Real-World Use Cases: Generative AI in Action for the Climate:
- Several projects illustrate generative AI’s impact. The Spherical DYffusion model, developed by the University of California San Diego and the Allen Institute for AI, predicts 100 years of climate patterns in 25 hours—25 times faster than traditional models—using GPU clusters instead of supercomputers. Showcased at NeurIPS 2024, it emulates atmospheric dynamics and is poised to simulate CO2 impacts, offering insights into long-term climate trendsÂ
- Google’s SEEDS model, published in Science Advances, generates large ensembles of weather forecasts at low cost, improving uncertainty quantification for extreme events like Hurricane Ian in 2022, aiding disaster preparedness.
- NASA’s use of AI to enhance satellite data analysis, as noted in a February 2025 Climate Cosmos article, improves monitoring of carbon emissions and deforestation, supporting global climate goals . These examples highlight AI’s practical contributions to climate resilience.
How Generative AI Is Enhancing Climate Forecast Accuracy and Speed:
Generative AI delivers breakthroughs in climate modeling:
- Reduced Computational Costs: Models like Spherical DYffusion run on accessible GPU clusters, democratizing high-performance simulations.
- Faster Model Training and Predictions: AI completes century-long projections in hours, compared to weeks for traditional models, accelerating research cycles.
- Greater Spatial and Temporal Resolution: AI captures fine-scale climate variations, improving regional forecasts critical for vulnerable communities.
- New Insights from Noisy Data: By reconstructing incomplete or noisy datasets, AI extracts actionable patterns, enhancing understanding of complex climate systems.
These advancements enable timely, precise forecasts, empowering decision-makers to act swiftly.
The Risks and Challenges of Using Generative AI in Climate Modeling:
Despite its promise, generative AI faces challenges. Data bias can skew predictions if training datasets underrepresent certain regions or climates, potentially misguiding policy. Model accuracy remains a concern, as generative outputs may overfit or produce unrealistic scenarios, requiring rigorous validation. Interpretability is another hurdle; AI’s “black-box” nature complicates understanding how predictions are derived, risking overreliance by non-experts. Additionally, the environmental footprint of training AI models is significant, with GPT-3’s training emitting 552 tons of CO2 and consuming vast energy and water resources . Ethical concerns, including data privacy and equitable access for developing nations, further complicate deployment.
The Future of Generative AI in Climate Research and Policy Making:
The future of generative AI in climate modeling is bright. Its integration with Earth system science will enhance holistic simulations, incorporating oceans, ice sheets, and biosphere interactions. Open-source AI models, as advocated at NVIDIA GTC 2025, could foster global collaboration, enabling researchers in resource-constrained regions to contribute to climate solutions . AI’s role in policy will grow, providing real-time data for international agreements like the Paris Accord. Innovations in energy-efficient AI training, such as scheduling during low-carbon grid periods, will mitigate environmental impacts, ensuring sustainability
Generative AI and Climate Modeling: A Critical Partnership for the Planet’s Future:
Generative AI is reshaping climate modeling, offering faster, more accurate, and accessible tools to combat climate change. From high-resolution simulations to extreme weather predictions, its applications promise to enhance resilience and inform global strategies. Yet, challenges like environmental costs, data bias, and interpretability demand careful management. As the climate crisis intensifies, policymakers, researchers, and tech leaders must collaborate to harness AI’s potential responsibly, ensuring it serves as a cornerstone for a sustainable future. The time to act is now—leveraging generative AI could redefine our fight for the planet.